Nobel prize in Physics: They trained their machines to imitate the human brain
New Delhi: Machine learning is a key aspect of artificial intelligence, but what do machines “learn”, and how? They cannot think, understand or know things the way humans do, but they can be trained to process information using a physical setup that is similar to that in our brains. Just as our brain cells, called neurons, communicate across the network connecting them, machine learning utilises an artificial neural network that emulates these functions.
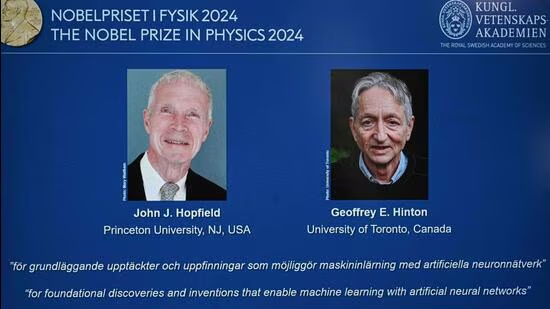
The winners of this year’s Nobel Prize in Physics, announced on Tuesday, are pioneers who developed early artificial neural networks. American physicist John Hopfield, 91, and British-Canadian computer scientist Geoffrey Hinton, 76, used fundamental concepts of physics to develop their ideas.
Hopfield, currently with Princeton University, created an artificial structure that can store and reconstruct information. Hinton, currently with the University of Toronto, worked on Hopfield’s ideas decades ago to create a machine that generates new data based on data fed into it. Hinton, of course, is a very familiar name. Known as the “godfather of artificial intelligence”, he has famously expressed concerns about AI surpassing human intelligence one day; he quit Google so that he could “talk about the dangers of AI”.
Hopfield’s network
The brain’s neurons communicate with one another through the spaces between them, called synapses. This has been understood since the 1940s, and it was inevitable that researchers would try to recreate the network at an artificial level.
The objective was to create “nodes” that would perform the function of neurons, while the connections between them would perform the function of synapses.
Although interest flagged towards the late 1960s, it was revived in the 1980s with new breakthroughs, including by Hopfield and Hinton.
Hopfield built an artificial neural network using his knowledge of atomic spin. The spin of an atom, which effectively makes it a magnet, affects the spin of adjacent atoms, and Hopfield used the physics that dictates these connections.
When an image is fed into the Hopfield network, followed by another, it can keep changing the colours of pixels in the latter image until the original image is recreated.
The method allows several pictures to be saved at the same time, and the network can differentiate between one image and another.
In effect, the Hopfield network can recognise information that it has been fed, in this case an image. But how does it interpret what that image depicts? That is where Hinton’s work comes in.
Hinton’s machine
Humans can recognise something they have never seen before because they associate it with known examples. To use an allegory cited by the Royal Swedish Academy of Sciences, a child can identify a dog or a cat even when they see it for the first time, because they associate its appearance with that of other dogs and cats they have seen earlier.
Could machines learn to process patterns in a similar way? This was a question Hinton, then at Carnegie Mellon University, was pondering. Starting from the Hopfield network, Hinton and his colleague Terrence Sejnowski used concepts of statistical physics to take the idea to a new level.
Take another allegory from the Academy. It is impossible to track all the molecules in a certain amount of gas, but one can analyse them collectively to determine various properties of the gas. Statistical physics uses the laws of probability to analyse various states in which the molecules in a gas (or the components in any system) can jointly exist. There is an equation to analyse this, developed in the 19th century by the physicist Ludwig Boltzmann, and Hinton build his network using this equation. When he published his method in 1985, he named it the Boltzmann machine.
The Boltzmann machine uses the knowledge it gains from patterns fed into it to generate new patterns. It learns from examples it has been given, and can recognise familiar traits in information it has not previously seen, just as a child recognises a new dog because it knows what a dog looks like.
Over the years, Hinton has kept updating the machine to make it more and more efficient.
Machine learning today
Machine learning’s areas of application are rapidly expanding. In recent years, it has been used to calculate and predict the properties of protein molecules and to work out which new versions of a material will be most efficient in solar cells.
“There’s been a surge of machine learning methods in the domains of computational chemistry and materials. Artificial neural networks are among the most popular of these,” said Anatole von Lilienfeld whose work at the University of Toronto’s material science department relies on machine learning.
“The Boltzmann machine was a trailblazer. In terms of chemistry and materials this matters because given sufficient training examples, properties and behaviour of novel chemicals and materials can be predicted without a costly experiment,” he said.
Disclaimer: The copyright of this article belongs to the original author. Reposting this article is solely for the purpose of information dissemination and does not constitute any investment advice. If there is any infringement, please contact us immediately. We will make corrections or deletions as necessary. Thank you.
Title:Nobel prize in Physics: They trained their machines to imitate the human brain
Url:https://www.investsfocus.com